DIFFERENTLY
Changing the traditional drug discovery process
is opening new pathways beyond rational design.
Unlike other drug discovery platforms that rely solely on simulations and theoretical models, MolecuLern harnesses the immense power of real empirical data.
- Hundreds of “tech-bio” companies are using AI to replicate the traditional drug development process; not change it. Computing power is being employed to do tests quicker or with improved accuracy. This isn't AI per se; just better use of computing power.
- MolecuLern was trained as a true AI tool, thus removing the need to perform certain work in the traditional development process because the AI engine is only generating molecules with certain characteristics; it has been taught how to do so.
- MolecuLern is being fed proven proprietary data; not consuming data points that have yet to be understood or assigned a value. This includes wet lab data and a high quality compound library. The quality of what MolecuLern is trained on is a key differentiator.
- MolecuLern is leveraging AI to learn how to reduce/eliminate steps and factors that add time, complexity and risk to early stages of drug development.
- MolecuLern is getting better hits on compounds because:
- We trained it to know what to look for.
- We need fewer hits (equates to shorter time) because we don't screen everything. We only screen for known properties of value.
- The more the MolecuLern process runs new searches and is fed resulting pre-clinical test data, the more it learns how to create compounds with stronger drug-like properties.
- For companies looking to reduce development time by up to 90% while increasing the probability of success, MolecuLern is a proven solution.
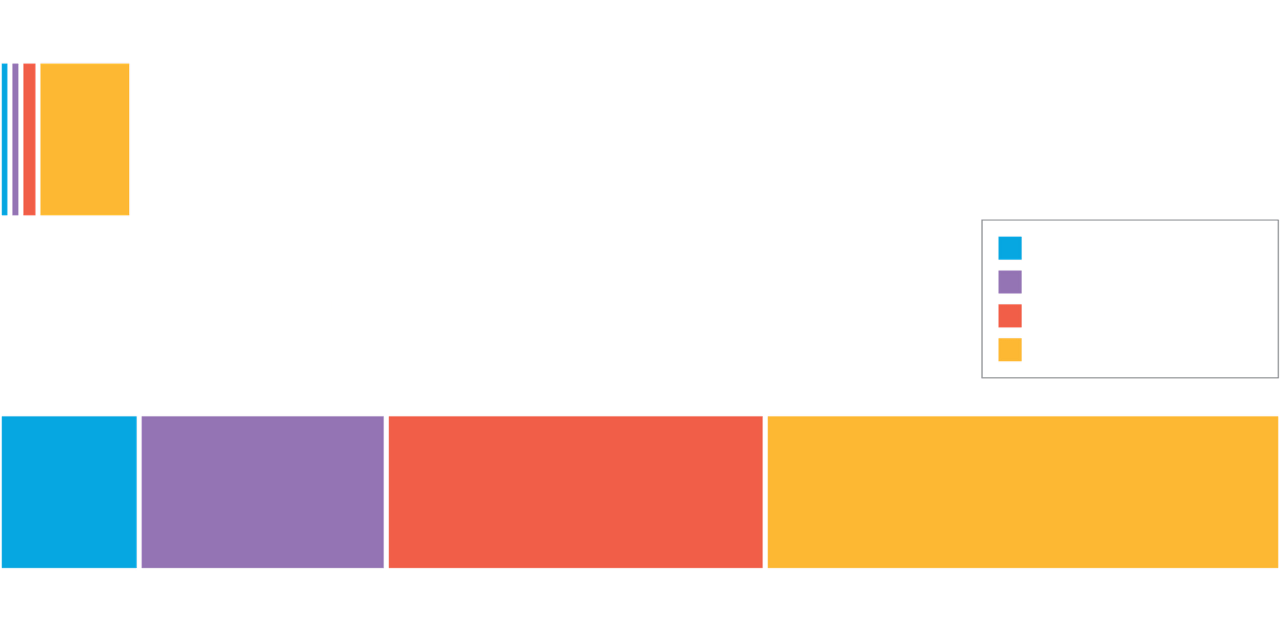